Imagine having a magic ball to foresee things about your business. What would you do?
Maybe you’d like to know exactly what your users are looking for, so you could better serve them. Or, you’d want to know what prices will bring you the most profit on a given day. Perhaps, you’d like to catch the ill-intentioned users even before they do any harm to you and others on your platform.
What if I tell you it is possible? Shared economy leaders use machine learning for eCommerce and p2p marketplaces to do these and many other great things that have a direct impact on their success.
In this article we are going to explore three major ways to use machine learning in the p2p marketplace:
- Predicting what your users will like
- Predicting the best prices
- Predicting and preventing fraud.
And we support each use case with real-world examples from market leaders.
Let’s dive in.
1. Predicting What Your Users Will Like
Personalization of search and recommendations is among the most common ways of applying machine learning to the p2p marketplace. Marketplaces with heterogeneous offerings are the ones that benefit the most from these solutions.
If your marketplace offers unique goods or services then you know that it is both a blessing and a curse. It is always good to be different and offer one-of-a-kind things, but what a complicated task it becomes for a buyer to sort through the endless options. And the more you have to offer, the harder it is to find what one is looking for.
Etsy and Airbnb, among others, are faced with this challenge every day. And both of them have millions of unique listings! Top that with unique preferences of buyers and, sometimes, even sellers (in the case with Airbnb hosts have preferences for the types of guests they want) and you get the idea.
You can imagine that these guys would have never made it if they hadn’t invented systems that help users find exactly what they want.
And they did. First, those systems were based on hard-coded rules and they took fewer factors into account. As a result, it was impossible to personalize search results for each user.
However, then they’ve started experimenting with machine learning and, at the same time, collecting vast amounts of data about their users. Both Airbnb and Etsy (among others) train machine learning systems on their big data to become better and better at predicting what their users are really looking for.
According to Mike Curtis, VP of engineering at Airbnb, their machine learning system takes more than a hundred signals (ranking factors) into account. Based on the ML algorithms, the system assigns weights to these factors and comes up with predictions.
“For example, what kind of setting is it in? What kind of decor is in the house? These are things Airbnb can use to feed into the model to come up with a better prediction of which listings to show you first.” Mike Curtis.
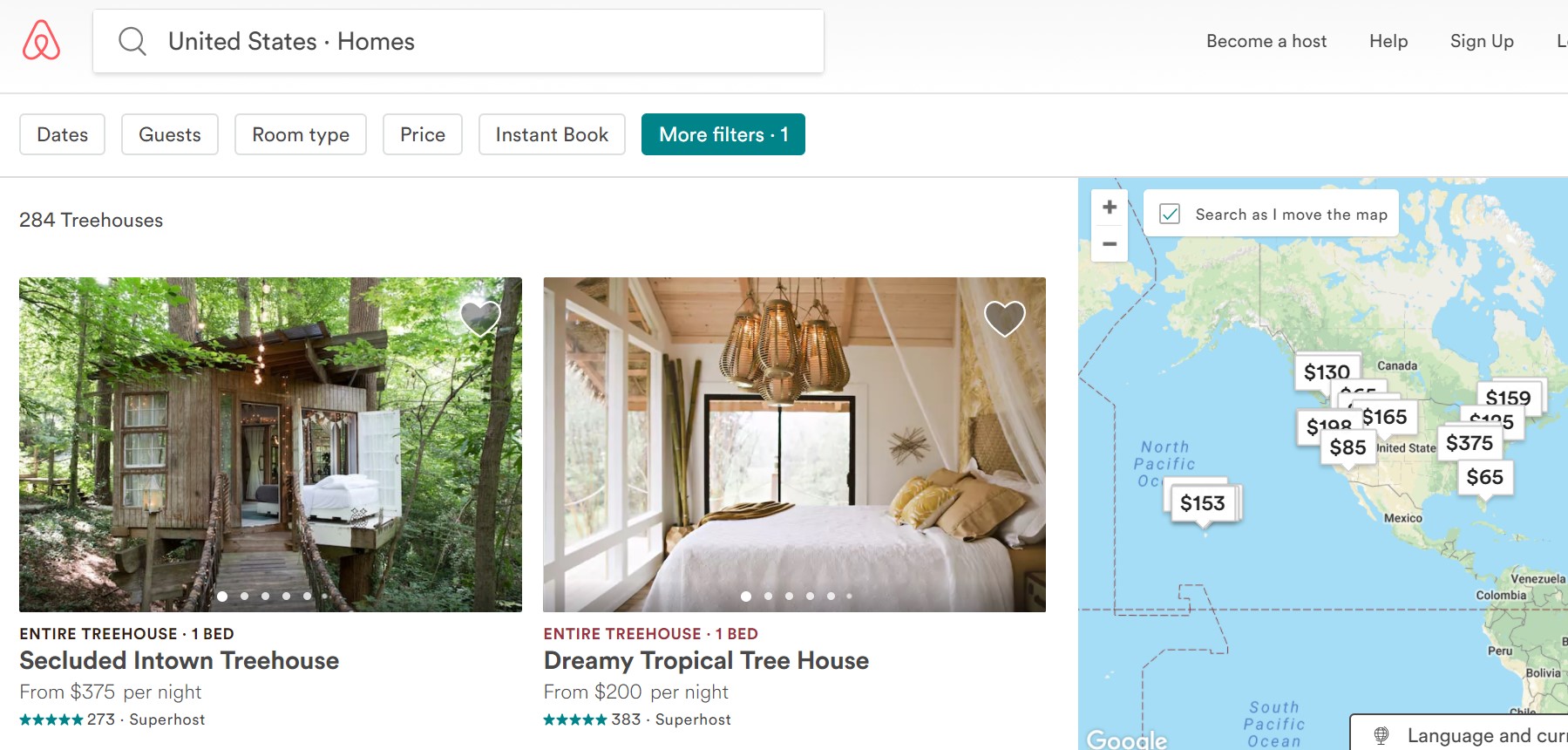
Etsy follows an analogous strategy. They also use machine learning to predict what their users will like, based on their past behavior, as well as on the behavior of users with similar profiles.
Etsy’s search results and recommendations are based on:
- Your preferences
- Preferences of users like you
- Shops with similar profiles to the ones you shopped at.
All of this makes their worldwide marketplace with over 40 million unique listings more personal and ‘discoverable’ for their buyers.
2. Predicting The Best Prices
In regard to pricing, there are two problems:
- How to price unique goods and services?
- How to adjust prices to market changes?
Let’s see what each of them means and how p2p marketplace machine learning solutions can help.
Pricing Unique Goods and Services
Not all shared economy players have to deal with the first problem, as not everyone offers unique listings. For example, Uber doesn’t struggle so much with it, as they offer a generic taxi-hailing service. And it’s usually quite easy to figure out market prices for generic products and services (e.g., used goods or cleaning services). However, it is very different for those who offer one-of-a-kind things like hand-made crafts or lodging in people’s homes.
How should one determine what a night in his guest room is worth?
Dan Hill, Product Lead at Airbnb, said that their hosts used to struggle with this question a lot. As a result, the company had a huge drop-off in registration at the point when hosts had to determine the price of a stay in their houses.
That is why Airbnb engineers have launched a machine learning feature called Price Tips. It is a mathematical model that predicts how likely your listing is going to be booked at specific prices on specific dates. And, based on this model, you will get price suggestions that increase your chances of getting booked, while earning as much as you can.
This model takes three groups of factors into account:
- Location
- Likeness
- Recency.
We all know that location is very important when choosing your accommodation in a foreign city. Sometimes we are willing to pay more for proximity to the center or to some recreational areas (e.g. beaches). Other times, we will pay the surplus to stay in a nice neighborhood. Price Tips take into consideration all of these location factors.
Then the system compares your accommodation to others listed on the platform by a number of factors, like room and property type, as well as reviews and capacity.
In addition to that, time factors also play a big role in pricing. I am talking about seasonality, availability, and how far off each available date is.
So the Price Tips ‘machine’ will account for all of these and even more factors in real-time and show you a price range that is best for you.
Adjusting Prices in Real-Time
The price that works for a business today might not be the one that’ll work for you tomorrow. As seasons, competition intensity and demand change so should your prices.
If they don’t, you and your sellers will lose profits. This may happen either because some of your listings are overpriced and no one is buying them, or because they are underpriced at peak demand times (e.g. some big event in your city).
I’d like to continue the Airbnb example because it does more than just help hosts with the initial price set up. Price Tips also support them with ongoing price recommendations. So the system constantly monitors all of the mentioned above factors and notifies hosts when price adjustments have to be made.
And Airbnb is not the only platform that’s faced with pricing challenges. Although Uber doesn’t have the problem of putting a price tag on something unique and personal, they still struggle with figuring out their responsive pricing.
Uber’s challenges are:
- Being profitable with extremely low or even negative profit margins (subsidized trips)
- Increasing driver efficiency at peak demand.
You’ve probably heard that Uber hasn’t been able to break even yet. Notably, these negative profit margins from subsidized trips account for the majority of their losses. So, given Uber’s scale, even the slightest changes in their prices can make a huge impact on the bottom line.
Uber has also noticed that drivers become inefficient during the demand peaks, as requests become spread out, so drivers make fewer trips per hour. And this is bad for everyone, including riders who have to wait longer.
To overcome both of these challenges Uber has been using its Dynamic Pricing (Surge Pricing) mechanism that increases prices in response to increased demand. This has helped with both improving the revenues and driver efficiency (as requests become denser). But, apparently, it doesn’t solve all of their problems.
This year, they’ve taken it a step further and introduced the so-called Route-Based Pricing. It is a machine learning solution that predicts how much riders will be willing to pay depending on the destination, time of day, and location.
With this new pricing strategy, a passenger going to some wealthy area may be charged more, than someone traveling to a poor neighborhood, given the same mileage and time to travel.
Quite a controversial move, let’s wait and see how this works out…
3. Predicting and Preventing Fraud
“To share is to trust. That, in a nutshell, is the fundamental principle.” Roland Berger, ‘Think Act, Shared Mobility’.
A shared economy is largely about trusting complete strangers with things of value to you (your money, house, car, pet, etc.). Some even refer to trust as a currency of collaborative consumption.
There are many ways to make your marketplace trustworthy and secure. And the reputation system is among the most popular crowdsourced solutions that most p2p platforms use nowadays. It incorporates ratings and reviews that each user accumulates while being active on a platform.
While this is a great tool that definitely helps us in choosing who to deal with, it doesn’t solve the trust problem in its entirety. One of the biggest disadvantages of a reputation system is that it only prevents fraud from reoccurring, but does nothing to prevent it from happening in the first place.
Thus, the shared economy leaders started building custom systems that help them predict fraud and take timely measures to prevent it from happening.
And here is in summary how machine learning makes it possible:
“By building models based on historical transactions, social network information, and other external sources of data, machine learning algorithms can use pattern recognition to spot anomalies, exceptions, and outliers. This helps detect and prevent fraudulent transactions in real time, even for previously unknown types of fraud.” HBR article ‘8 Ways Machine Learning Is Improving Companies’ Work Processes’.
Airbnb is facing all types of online fraud on a daily basis:
- Payment fraud
- Fake listings
- Spam messages
- Account hijackings.
Once they’ve even been infected with a so-called ‘time travel’ bug that caused guests to make bookings in the past. They were able to revert it in 24 hours with a machine learning solution.
Later they’ve built an ML-based fraud prevention service. Eric Levine, Software Engineer at Airbnb, says this service is able to discover fraudulent patterns, detect risks, and act on them.
How to Apply This To Your Business
Being a marketplace owner, your job is to facilitate the best buyer-seller matches and smooth transactions. In addition to that, you also need to optimize for revenue so that you and your sellers could stay in business.
Using machine learning in a peer-to-peer marketplace can help you do this job in the most efficient manner.
Aggregate data about your users’ preferences and use machine learning systems to make accurate predictions of what your users are looking for.
Leverage ML to predict the best prices for unique goods and services, as well as to adjust your prices to the ever-changing market conditions.
Stop malicious actors in their tracks with a system that recognizes fraudulent patterns and takes necessary actions.
And these are just a few reasons to develop a p2p marketplace with machine learning technology.
I hope this article was useful!
Don't hesitate to hire a peer-to-peer marketplace specialist!
Contact UsRate this article!
5
Comments (0)