Envision a world where your customer service never sleeps, effortlessly handling inquiries with the charm of human interaction. This isn't a distant dream—it's the reality with AI chatbots.
For business owners eyeing digital evolution, adding an AI chatbot to your website is more than an upgrade; it's a game-changer. These intelligent agents not only enhance customer experience but also streamline operations, offering a seamless blend of efficiency and engagement. Discover why integrating an AI chatbot could be your strategic move towards unparalleled customer satisfaction and operational excellence.
In this article, we will figure out if it suits your business and how to make use of it. The Greenice team has extensive practical experience in this area, having developed chatbots using GPT models and other AI tools. We are here to share our knowledge, guide you through the development process, and provide an overview of the pros and cons, as well as an estimate of the cost of creating an AI chatbot.
What is an AI chatbot and how did generative AI change everything?
An AI chatbot is a software application that engages in conversation with users through natural language, thanks to artificial intelligence (AI).
Let’s look at chatbots’ evolution:
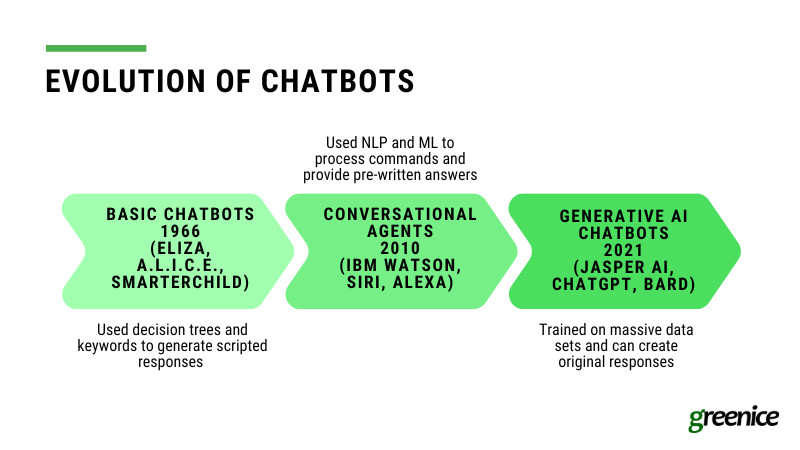
Upon ELIZA's 1966 debut, chatbots operated on a rule-based system, relying on predefined rules and scripts to respond to specific keywords or phrases, offering efficiency for straightforward tasks but lacking flexibility. As technology evolved, AI-powered bots emerged, utilizing machine learning (ML) and natural language processing (NLP) to grasp the context and nuances of conversations. These AI chatbots acquired the ability to learn from past interactions, improving their responses over time, making them more suited for complex queries and personalized interactions.
The journey from rule-based systems to AI chatbots has seen the integration of conversational AI (IBM Watson release in 2010), focusing on human-like interactions, and generative AI (Jasper AI release in 2021), which excels in creating original content from patterns in data. Conversational AI is tailored for customer service by providing pre-written responses that in a way mimic basic human conversation. On the contrary, generative AI pushes boundaries in creativity and is capable of generating more human-like responses as well as content creation.
Chatbots can be integrated into a variety of applications and services, including customer service, personal assistants, and educational tools.
Why build an AI chatbot?
Embracing AI chatbots isn't just an upgrade; it's a strategic move for any business looking to thrive in the digital age. Let’s look at some reasons for chatbot adoption:
- Workforce Transformation: Automate routine tasks to free up your team for more complex, strategic initiatives, shifting the focus from mundane to innovative work.
- Strategic Insights: Leverage the chatbot's ability to analyze vast data sets, uncovering market trends and customer preferences that inform better decision-making.
- Enhanced Customer Service: Provide highly personalized interactions, making customers feel valued and understood, which boosts loyalty and enhances the perception of your products and services.
- Cost Efficiency: Substantially reduce expenses associated with repetitive tasks, translating technological advancement into a strategic financial decision.
- Dynamic Operations: Ensure your business is responsive, agile, and cost-effective, meeting the evolving needs of the market and positioning your company as a leader in innovation.
Conversational AI vs Generative AI
Conversational AI and Generative AI are two distinct approaches to creating chatbots.
What is Conversational AI?
Conversational AI is technology designed to simulate human-like interactions, focusing on specific tasks within a limited data set for direct customer service responses. It integrates with business systems for detailed, personalized answers but is limited by a rigid conversational flow with predefined responses. This technology requires regular updates to improve understanding and adapt to customer interactions, demanding high maintenance effort to stay effective.
What is Generative AI?
Generative AI, meanwhile, refers to AI systems that can generate new content based on the data they have been trained on. This includes creating text, images, music, and more. In the context of chatbots, generative AI can produce responses that weren't explicitly programmed into the system, allowing for more dynamic and varied interactions with users.
How do they differ?
Conversational AI identifies user intent and retrieves the closest match from a set of predefined answers, streamlining user interactions. In contrast, Generative AI, leveraging NLP (as well as databases and instructions), not only comprehends user requests but also crafts original responses, offering interactions that more closely mimic human conversation. This technology excels in understanding and responding to inquiries with a more nuanced and personalized touch than its Conversational AI counterpart.
On the other hand, Conversational AI systems like AWS Lex and IBM Watson, having been in the market for a considerable time, are recognized for their maturity, reliability, and predictability, thanks to extensive testing and research. Conversely, Generative AI is a newer frontier, still in its exploration phase with much to refine and optimize. This novelty contributes to its current status as a less reliable and predictable technology, but with potential for future advancements.
Pros and cons of Conversational AI
Conversational AI chatbots blend predefined rules with AI to offer distinct advantages and face specific challenges:
Pros
- Adherence to Instructions: They reliably follow set instructions, ensuring accurate and consistent information delivery.
- High Security and Compliance: Built to strictly adhere to data protection laws, making them suitable for sensitive industries.
- Consistent Quality: Provide unwavering service quality 24/7, without the variability seen in human performance.
- Efficient Scalability: Capable of handling numerous inquiries simultaneously, ideal for managing high volumes of customer interactions.
Cons
- Rigid Conversations: They are very limited in terms of understanding user intents and can only give pre-scripted responses, resulting in a lack of flexibility in handling unique or complex queries.
- Limited Personalization: Struggle to tailor interactions based on individual user history or preferences.
- Struggle with Subtleties: Often fail to grasp the nuances of human communication, such as emotional undertones or sarcasm.
- User Frustration Risk: The inability to deviate from scripted paths may lead to user dissatisfaction in some interactions.
Pros and cons of Generative AI
Generative AI chatbots, powered by advanced AI models like GPT (Generative Pre-trained Transformer), offer a leap forward in how machines understand and interact with humans. These chatbots have their own set of advantages and drawbacks:
Pros
- Advanced Understanding of Human Speech: Generative AI chatbots are equipped with the capability to comprehend complex human speech and requests with a higher degree of accuracy, thanks to their training on vast datasets of human language. This allows for more natural and fluid conversations.
- Dynamic Response Generation: Unlike their rule-based counterparts, these chatbots can generate responses on the fly, allowing for more engaging, varied, and contextually relevant interactions that aren't limited to pre-defined scripts.
- Continuous Learning and Improvement: With each interaction, generative AI chatbots have the potential to learn and adapt, improving their accuracy and the relevance of their responses over time.
- Creative and Flexible Interactions: They can creatively address queries and even generate content, such as writing, artwork, and more, making them versatile tools for both customer service and creative applications.
Cons
- Security and Compliance Risks: Generative AI chatbots may pose risks in terms of security and compliance, as their open-ended nature can lead to the generation of unexpected or inappropriate content, making them less suitable for regulated industries without significant oversight.
- Tendency to Hallucinate: These chatbots can "hallucinate" or generate false or irrelevant information, as their responses are not always based on factual data but rather on patterns learned during training. This can lead to misinformation if not carefully managed.
- Potential for Bias: Like all AI models, generative AI chatbots can inherit biases present in their training data, leading to responses that could be seen as inappropriate or offensive, requiring rigorous testing and refinement to mitigate.
- Complexity in Integration: Integrating generative AI chatbots into existing systems can be complex and challenging, requiring robust APIs and understanding of both the AI model and the host system's architecture.
Is it possible to combine these technologies for quality and optimization sake?
Combining Conversational and Generative AI can indeed make a chatbot more powerful, leveraging the structured interaction and reliability of Conversational AI with the creative and dynamic response generation of Generative AI. This hybrid approach allows for chatbots that are not only efficient in handling routine queries but also capable of engaging in more complex, personalized conversations.
To achieve this, developers can integrate Generative AI models within a Conversational AI framework, using Generative AI to enrich the chatbot's responses and Conversational AI to guide the interaction flow and maintain relevance to the user's queries. This combination offers the best of both worlds, ensuring that chatbots are both helpful and engaging, capable of meeting a wide range of user needs. And we speak about it from our experience, as we worked on a project which required this kind of solution.
How to minimize weaknesses of generative AI chatbots?
To overcome risks associated with using AI chatbots, companies are adopting various effective strategies:
- Enhance Security and Content Management: By deploying content filters and security guardrails, companies can block inappropriate content and prevent chatbots from accessing unreliable third-party data. This ensures data integrity and protects users from misleading information. Additionally, transferring complex queries to human operators helps maintain reliability and user trust.
- Maintain Data Quality and Operational Integrity: Regularly upgrading data quality and setting clear operational boundaries for AI chatbots are crucial. This includes preventing "model drift," defining what chatbots can and cannot do, and conducting thorough risk assessments to prepare for potential issues. Engaging experts early in the development process helps identify and mitigate risks effectively.
- Research and Continuous Improvement: Investing in research to reduce chatbot "hallucinations" and improve accuracy, along with applying common sense to AI operations, are key to enhancing chatbot performance. Building larger, more intelligent models and enabling self fact-checking mechanisms can significantly boost reliability.
- Transparency and User Support: Being transparent with users through clear disclaimers about interacting with AI, coupled with providing options to escalate to human support, ensures a user-friendly experience. Regularly monitoring chatbot performance and having an "emergency brake" to address adverse outcomes are essential for maintaining service quality.
- Guardrails Evaluation: Identifying and continuously evaluating the effectiveness of implemented guardrails, like controls to focus chatbot responses and reduce errors, ensures the chatbot remains effective and relevant to user needs over time.
- Use Retrieval-Augmented Generation (RAG): Enhance chatbot responses by integrating RAG, which combines pre-trained models with information retrieval to access and use external data, like a company’s knowledge base or set of textbooks, for more accurate and detailed answers, especially beyond the chatbot's initial training data.
Examples of AI chatbots
Chatbots are transforming diverse industries such as eCommerce, marketing and sales, travel and hospitality, real estate, education, and HR management. However, high-stakes areas such as healthcare or finance could be risky for this new technology. Let's find out where AI chatbots can work best and look at successful examples.
eCommerce
Chatbots can assist customers in navigating web pages and providing personalized recommendations. However, it is recommended to set up a payment process outside the bot to avoid personal data breaches. For example, Shopify has integrated GPT-3.5-turbo with an AI shopping assistant in a Shop app. Now it makes personalized product recommendations for users.
Marketing and Sales
Chatbots collect and analyze customer feedback, track and nurture leads, and personalize messages. For example, our company, Greenice, uses a GPT-4-powered bot to consult clients on their projects, also offering time slots to book a call with our team.
Travel and Hospitality
Chatbots help travelers find necessary information without browsing multiple sites. For example, Expedia created a GPT-4-powered AI assistant that offers users personalized hotel recommendations.
Education
Chatbots fulfill mundane tasks like sharing timetables and monitoring progress. For example, Khan Academy uses GPT-4-powered AI assistant Khanmigo for education. It serves as both an assistant for teachers and a tutor for students.
HR
Chatbots relieve managers of answering repetitive questions and assist with pre-screening resumes and scheduling interviews. For example, Morgan Stanley has an AI chatbot with GPT-4. It helps personnel effectively use the company's database.
Social Media and Entertainment
While GPT models are great at processing human speech, they can also entertain people. For example, Snapchat integrated GPT-3.5-turbo with ‘My AI’ chatbot. The bot offers recommendations and entertainment, e.g., it can write poems for users.
Customer Service
Bots are for sure can be used to boost customer service. For example, Maruti Suzuki developed a WhatsApp Chatbot with DaveAI, providing informative conversations about their cars through an accessible platform.
What chatbot technology to choose?
As long as Conversational AI and Generative AI are two different approaches, they also use different technologies. Let’s look at the most popular and functional options for each approach.
Top conversational AI technologies for chatbots (Amazon Lex, IBM Watson)
- Amazon Lex: Utilizes the same technology as Amazon Alexa, providing chatbots with automatic speech recognition and natural language understanding for lifelike interactions. This technology is a part of Amazon Connect- a comprehensive platform for building chatbots as well as full-scale call centers.
- IBM Watson: Known for advanced natural language processing, Watson allows the creation of sophisticated conversational agents capable of complex interactions.
- Google Dialogflow: Features an intuitive interface and integrates with Google's AI services, making it easy to build chatbots that understand user intent across various platforms.
- Microsoft Bot Framework: Supports the development of bots that can interact across multiple channels, including websites and messaging platforms, using machine learning for smarter conversations.
- Rasa: An open-source framework focused on building chatbots with customized conversational abilities, perfect for developers needing deep system integration.
Top generative AI technologies for chatbots
- OpenAI’s GPT-4: GPT-4 is the latest iteration of the Generative Pre-trained Transformer series, known for its exceptional ability to understand context and generate coherent, diverse text based on minimal prompts.
Here is our article if you want to better understand the difference between GPT-3 vs. GPT-3.5-turbo vs. GPT-4. - Google’s LaMDA and PaLM: LaMDA (Language Model for Dialogue Applications) is designed specifically for conversational applications, offering nuanced and context-aware responses, making it particularly effective for complex dialogue systems. PaLM serves as the foundation for Bard, showcasing advanced conversational abilities.
- Hugging Face's BLOOM and XLM-RoBERTa: Hugging Face provides a wide range of capable language models, including BLOOM for multilingual understanding and generation, and XLM-RoBERTa for cross-lingual masked language modeling, enhancing chatbots' ability to interact in various languages.
- Nvidia's NeMO LLM: Neural Modules LLM focuses on optimizing language models for conversational AI, providing tools for building and deploying more efficient and scalable chatbot applications.
- Antropic’s Claude: An advanced language model known for its simplicity in integration and versatility in generating high-quality content across different domains, making it a solid choice for businesses looking to enhance their chatbot experiences.
- Meta's LLaMA 2 (Large Language Model Meta AI): An open-source model launched to enable developers to create customizable chatbots at a lower cost.
The trend towards open-source LLMs, such as BLOOM, XLM-RoBERTa, and LLaMA, reflects a growing movement among developers to build more tailored and cost-effective chatbot solutions. With the continuous evolution of these models, like Google's PaLM 2 which uses significantly more training data for improved performance, the future of chatbot development is geared towards more sophisticated, efficient, and versatile applications.
Features of an AI Bot
Chatbots may have different features depending on their purpose and industry. Let’s examine the most common features for all types of bots:
Basic features
1. Client widget
A client widget is a visible chatbot that appears on the screen after a certain action, such as opening a page or clicking on an activation button. Good chatbot UX should include the following attributes:
- Feel and sound natural and human-like to give the impression of a real conversation.
- Provide quick answers.
- Have a name and avatar.
- Have emoticons (emojis).
- Not leave a client stranded.
- Show a ‘typing’ message as if a real agent is typing the reply.
- Send different types of media files: (Gifs, videos, images, and audio messages).
2. Data collecting
If you want your chatbot to convert visitors into leads and clients, you need to consider what data to collect. Ask for the data that you need, like name, email, delivery address, preferences, order parameters, and feedback. Be careful not to ask for too much sensitive data. Ensure that data is securely transferred and stored, and check the regulations in your country or state. For example, platforms that provide services to European customers, have to comply with GDPR.
3. Integration with a CRM and other software
By integrating your chatbot with a CRM, you can automatically save lead information in a single place for future use. A chatbot can recognize returning customers and start the conversation from where it left off, providing personalized messages and recommendations based on previous requests and purchases.
4. Connecting to a human agent
Non-standard requests often require a human being's involvement. Provide an option to switch to a conversation with a live agent.
5. Agent panel
If your chatbot connects with human agents, the operators should be able to view queues and inquiries, choose from predefined answers, view previous chat history, and see their own KPI. The agent panel should be easy to use and have a smooth design because people will work with it for many hours a day.
6. Admin panel
An admin panel should be used to manage chatbot parameters. This can include:
- Role management: assigning roles and permissions to other team members
- Analytics: a dashboard of key metrics and different reports to see the effectiveness of the chatbot and agents
- Notifications: managing reminders and ads
- Subscribers: viewing a list of subscribers
- Chat history: the message history
- Flow editor: editing message flow and texts.
Extra features
1. Omnichannel integration
Modern brands should widen their online presence by being available on all possible customer channels, whether it be a website, mobile app, or messenger. Linking the chatbot with all these channels will ensure that all requests come to a single database and are processed in your CRM, decreasing the burden on client services.
2. Localization
According to CSA Research, 76% of online buyers prefer to make purchases in their native language, and 40% of shoppers refuse to buy from websites in other languages. Therefore, if you provide international services, using a multilingual chatbot is indispensable.
3. Recommendations
Platforms that provide a large variety of products can use chatbots to assist customers with their search. For example, Lidl created a sommelier chatbot that suggests the best wine based on the region, price, preferences, or composition of the meal.
Steps to build an AI chatbot from scratch
Custom development is the way to go if you want a chatbot tailored to your requirements and budget. Here are the steps for building a chatbot that meets the needs of its users and provides value to your business:
Discovery Phase
To ensure your chatbot project meets your expectations, it's crucial to start with a clear understanding of your goals. Ask yourself: What are your objectives? What specific use cases and scenarios do you foresee for the chatbot? This initial stage, known as the discovery phase, involves outlining your overarching aims for creating a chatbot and identifying the key functionalities it needs to support.
Through this process, you'll create a Software Requirements Specification (SRS), a comprehensive document that details every aspect of your forthcoming project. The SRS serves as a blueprint, guiding the development process to align with your vision and objectives, ensuring your chatbot fulfills its intended purpose effectively.
Choose Technology
Armed with your goals and a Software Requirements Specification (SRS), consulting an expert to choose your bot's technological foundation is a crucial next step. This decision involves determining whether to rely solely on conversational AI or to enhance your bot with generative AI through pre-trained models like GPT-4 or BERT. These models are celebrated for their advanced deep learning capabilities, enabling a profound understanding of and ability to generate natural language, making them indispensable for creating sophisticated and responsive AI chatbots that can engage in meaningful interactions.
Deciding on AI tech for your chatbot?
Consult our experts now!For Conversational AI - Design the conversation
Creating a bot with conversational AI using Amazon Lex involves drafting an interaction model to define the bot's tasks in a structure it can understand, including intents, sample utterances, slot names, values, and synonyms. This process starts with understanding user goals and crafting dialogs to achieve those. It's important to prototype your design, test with real users, and continually refine based on feedback. This iterative approach ensures your bot delivers natural and effective conversational experiences.
For Generative AI - Train (fine-tune) the Model with Domain-Specific Data
Tailor the chosen model to your specific needs by fine-tuning it with domain- and company-specific data. This involves training the model further on a dataset that is closely related to the topics and scenarios your chatbot will encounter. For example, if you're building a chatbot for eCommerce, you would fine-tune the model with typical shopping dialogues and terminologies.
To enhance this step, you can incorporate RAG (retrieval-augmented generation) into your chatbot's development. RAG allows you to augment the LLM prompt with additional, domain-specific context, enabling the model to deliver more accurate and relevant responses. This method enriches the model's understanding, ensuring that the chatbot can offer superior answers by leveraging the specialized knowledge embedded in your domain's data.
Develop an Interactive User Interface
Create a user interface that is intuitive and easy to use. The interface should facilitate smooth interactions between the chatbot and users, whether it's through text on a website, voice commands, or within a mobile app. Consider the platform's limitations and user expectations when designing the interface.
Incorporate Feedback Loops for Continuous Learning
Implement mechanisms for your chatbot to learn from interactions and improve over time. This could involve retraining the model periodically with new data collected from user conversations or using reinforcement learning techniques where the chatbot adjusts based on feedback received.
Test and Refine the Chatbot
Before full deployment, conduct thorough testing with real users to identify any gaps in understanding, responsiveness, or accuracy. Use this feedback to make necessary adjustments to the model, interface, or NLP processes.
Ensure Privacy and Compliance
Address any privacy concerns and ensure that your chatbot complies with relevant data protection regulations. This includes securing user data and being transparent about how data is collected and used.
Deploy and Monitor
Once your chatbot is refined, deploy it to your chosen platform. Monitor its performance closely, paying attention to user satisfaction, engagement levels, and any recurring issues. Be prepared to make iterative improvements based on ongoing insights and feedback.
Update Regularly for Relevance and Accuracy
Continuously update the chatbot’s knowledge base and algorithms to keep up with new information, changes in user behavior, or shifts in your business domain. This ensures that the chatbot remains helpful, accurate, and relevant over time.
At Greenice, we can help you with development right from the first steps. This includes the Discovery phase, planning, choosing the right model (or other technology), and creating a prototype. And of course, we can assist with integration, design, and development.
How much does it cost to build an AI chatbot?
To estimate the cost of building an AI chatbot, there are many factors to consider, including the cost of chat development itself and the price of the model used.
When it comes to developing a chatbot, it requires a lot of planning, design, tuning/training, front-end and back-end development, and testing. You'll need a team of programmers, designers, testers, and also a Team Lead and Project Manager.
The cost of development teams can vary greatly based on the team hourly rate and the time they will spend on your project. Development time depends on the complexity of your project and team experience while the rate depends on the team's reputation and location. Prices for development teams can start from $20-40 per hour in Asia and Africa, $30-50 per hour in Latin America and Eastern Europe, $75-100 per hour in Western Europe, and $90-150 per hour in North America.
However, opting for the cheapest team may lead to a longer project timeline, a bug-ridden product, and potentially extensive rework or a complete redo.
The price also depends on the technology you are using.
The cost of integrating GPT models is token-based, where 1,000 tokens approximate 750 words, leading to a monthly recurring expense. The prices start from $0.0005 per 1k input tokens/$0.0015 per 1k output tokens for the GPT-3.5-turbo model, increasing with the model's complexity and capabilities.
Amazon Lex pricing starts with a free tier for new users, offering up to 10,000 text and 5,000 speech requests per month for the first year. Beyond the free tier, prices for text requests start from $0.004 per request, and speech requests from $0.0065 per request. The service also charges for the Automated Chatbot Designer feature based on training time and the number of transcripts analyzed.
IBM Watson Assistant offers three pricing editions, ranging from a $0 Lite version to a $140 Plus version, with custom pricing available for enterprise needs. Additionally, a free trial of IBM Watson Assistant is provided, allowing users to explore its features before committing to a paid plan.
At Greenice, we have a lot of experience developing custom solutions, including AI-powered projects and custom chatbots. We can take care of your project from idea creation to after-launch maintenance. Based on our experience, development of the AI chatbot will cost from $10,000 for an MVP.
Our experience
At Greenice, we have extensive experience and worked with both conversational and generative AI. Here are a couple of cases.
ChatGPT-like chatbot for a website
We enhanced our website's customer support by integrating a GPT-4 powered chatbot. This advanced bot is equipped to advise clients on the company’s expertise, technologies, services, and pricing, and it can schedule live calls. It operates on three key components: a phrase library specific to Greenice, system context for behavioral guidelines, and functions for executing tasks like price estimation or call scheduling. By analyzing user queries for keywords within its library, the bot delivers precise, relevant responses, streamlining the customer experience.
You can try Greenice AI assistant on our website!
Amazon Lex bot for doctor booking
We developed a chatbot for an online clinic, utilizing Amazon Lex for chatting and Amazon Connect for calls. The chatbot enables users to book doctor’s appointments and check symptoms, offering the option to respond with preset answers or custom text. When necessary, it can connect to a human agent. Using conversational AI, the bot analyzes messages to provide relevant responses. Additionally, it collects client feedback post-interaction, enhancing the user experience and service quality.
Future outlook for chatbots
Generative AI will most likely become a basis for all chatbots. With ongoing enhancements in this technology, chatbots are expected to deliver not only more contextually relevant responses but also understand and react to human emotions more accurately.
This evolution will likely mitigate existing challenges related to security, precision, and comprehension. Furthermore, as natural language processing and speech recognition technologies become more refined, future chatbots are expected to facilitate voice interactions that are seamless and intuitive, making the use of buttons for queries and responses a thing of the past. This shift will enable a more engaging and natural conversational experience, akin to human interaction, thereby enhancing user engagement and satisfaction.
And users seem to be ready for these changes. With 90% of adults using voice assistants in 2022 people will swiftly adapt to new audio abilities of the chatbots.
Unlock the potential of AI chatbots with our expert team
Contact usRate this article!
4.5
Comments (0)